PIs: Hen-Hsen Huang, Lun-Wei Ku, and Cheng-Te Li
Gaining awareness of people's emotions, understanding their concerns, and responding to their psychological needs are essential for an intelligent agent to establish deep collaboration with human beings. While large language models have made significant progress in recent years, it remains challenging for machines to provide long-term companionship by delving into people's deep concerns and offering appropriate responses to meet their needs. This project aims to develop empathetic reaction modeling in more realistic scenarios, where the intelligent agent is expected to recognize people's emotions and respond accordingly during multi-round, long-term, multimodal interactions.
To facilitate progress towards this goal, multimodal long-term empathy analysis plays a crucial role in fostering meaningful and more enjoyable communication. As illustrated in Figure 1, our framework enables the agent to have multimodal perception capabilities for gathering external information not only from the user directly interacting with the agent but also from world knowledge and social media over time. Furthermore, the agent will be equipped with long-term working memory to track empathetic transitions of external information. Most importantly, an inference mechanism will be integrated into the working memory to ensure logical consistency among all information and knowledge. Finally, a reaction module will generate responses based on the current state.
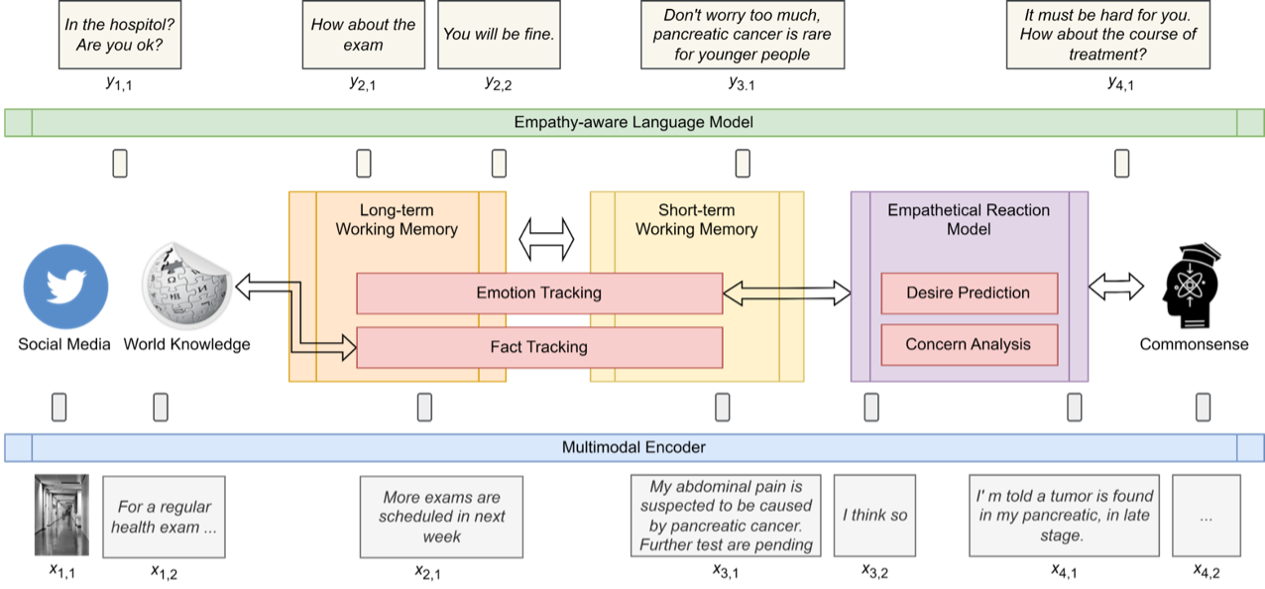
The research challenges of this project can be summarized as follows:
- Existing research on empathetic analysis primarily focuses on unimodal scenarios. Addressing the interplay among textual, visual, and graph-based information from an empathy response perspective requires a novel framework that takes advantage of large language models, image encoders, and graph neural networks. In this project, we will propose a universal representation encoder for various multimodal data types. Moreover, the encoder will be pre-trained with dedicated self-supervised tasks to make the representations empathy-aware.
- Social networks are crucial media modes that embody relationships among people in a society. The changes in an individual's or a society's social network over time represent alterations in personal relationships, leading to emotional and empathetic effects. In this project, we will explore temporal graph networks to capture the dynamics of a social network and extract underlying empathetic significances.
- To maintain multi-round long-term consistency during human-agent interactions over time, sophisticated manipulation of the agent's working memory is necessary. The working memory can be tightly integrated with the neural network model or function separately as an external information retrieval system. Furthermore, the content representation in the working memory can be either symbolic or distributed. While distributed representation condenses semantic information into dense vectors, symbolic representation capitalizes on logical inference. This project will propose a framework for long-term working memory manipulation that utilizes both types of representations.
- World knowledge and commonsense play crucial roles in ensuring the logical consistency of the working memory. It has been reported that large language models may sometimes produce results containing inaccurate or false information. When the agent perceives an entity, it can obtain information about that entity by consulting a world knowledge database (e.g., Wikidata). Conversely, a commonsense knowledge base (e.g., Atomic) provides information about the cause or effect of an event. Both types of information are essential to prevent the agent from behaving illogically. Exploiting external knowledge resources for a large language model is also a very hot research topic in its own right.
- As an initial investigation into this complex problem, we will establish a dataset and evaluation guidelines. Our dataset, intended to be a public research resource, will be built using materials without ethical concerns. However, collecting raw materials, such as logs of human-to-human or human-to-robot interactions, is challenging, especially when considering privacy issues. Additionally, an automatic approach to performance evaluation in our multi-round scenario has yet to be explored.
Our project addresses a fundamental open problem in natural language processing, with outcomes that have wide-ranging applications across various research areas. These include, but are not limited to, dialogue systems, argument mining, sentiment analysis, lifelog mining, and summarization. By uncovering the emotions and desires behind the text, our approach facilitates a deeper understanding, pushing the boundaries of natural language processing. Notably, the long-term consistency property derived from our methodology enables intelligent systems to perform tasks involving extended-length or long-duration data more effectively. Such tasks remain challenging for even the most advanced large language models.