Decoding the genome of bloodsucking midge Forcipomyia taiwana (diptera: Ceratopogonidae): Insights into odorant receptor expansion
Insect Biochemistry and Molecular Biology, May 2024
Ming-Der Lin, Chia-Hsien Chuang, Chih-Hsin Kao, Shu-Hwa Chen, Szu-Chieh Wang, Ping-Heng Hsieh, Guan-Yu Chen, Chun-Chia Mao, Jeng-Yi Li, Mei-Yeh Jade Lu, Chung-Yen Lin
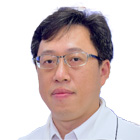
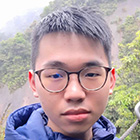
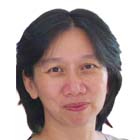
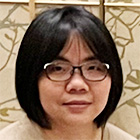


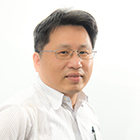
Abstract
Biting midges, notably those within the Ceratopogonidae family, have long been recognized for their epidemiological significance, both as nuisances and vectors for disease transmission in vertebrates. Despite their impact, genomic insights into these insects, particularly beyond the Culicoides genus, remain limited. In this study, we assembled the Forcipomyia taiwana (Shiraki) genome, comprising 113 scaffolds covering 130.4 Mbps—with the longest scaffold reaching 7.6 Mbps and an N50 value of 2.6 Mbps—marking a pivotal advancement in understanding the genetic architecture of ceratopogonid biting midges. Phylogenomic analyses reveal a shared ancestry between F. taiwana and Culicoides sonorensis Wirth & Jones, dating back approximately 124 million years, and highlight a dynamic history of gene family expansions and contractions within the Ceratopogonidae family. Notably, a substantial expansion of the odorant receptor (OR) gene family was observed, which is crucial for the chemosensory capabilities that govern biting midges' interactions with their environment, including host seeking and oviposition behaviors. The distribution of OR genes across the F. taiwana genome displays notable clusters on scaffolds, indicating localized tandem gene duplication events. Additionally, several collinear regions were identified, hinting at segmental duplications, inversions, and translocations, contributing to the olfactory system's evolutionary complexity. Among the 156 ORs identified in F. taiwana, 134 are biting midge-specific ORs, distributed across three distinct clades, each exhibiting unique motif features that distinguish them from the others. Through weighted gene co-expression network analysis, we correlated distinct gene modules with sex and reproductive status, laying the groundwork for future investigations into the interplay between gene expression and adaptive behaviors in F. taiwana. In conclusion, our study not only highlights the unique olfactory repertoire of ceratopogonid biting midges but also sets the stage for future studies into the genetic underpinnings of their unique biological traits and ecological strategies.
A Study on Incorporating Whisper for Robust Speech Assessment
IEEE International Conference on Multimedia and Expo (ICME), July 2024
Ryandhimas E. Zezario, Yu-Wen Chen, Szu-Wei Fu, Yu Tsao, Hsin-Min Wang, and Chiou-Shann Fuh
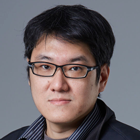
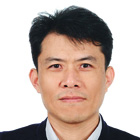
Abstract
This research introduces an enhanced version of the multi-objective speech assessment model--MOSA-Net+, by leveraging the acoustic features from Whisper, a large-scaled weakly supervised model. We first investigate the effectiveness of Whisper in deploying a more robust speech assessment model. After that, we explore combining representations from Whisper and SSL models. The experimental results reveal that Whisper's embedding features can contribute to more accurate prediction performance achieved by MOSA-Net+. Moreover, combining the embedding features from Whisper and SSL models only leads to marginal improvement. As compared to intrusive methods, MOSA-Net, and other SSL-based speech assessment models, MOSA-Net+ yields notable improvements in estimating subjective quality and intelligibility scores across all evaluation metrics in Taiwan Mandarin Hearing In Noise test - Quality \& Intelligibility (TMHINT-QI) dataset. To further validate its robustness, MOSA-Net+ was tested in the noisy-and-enhanced track of the VoiceMOS Challenge 2023, where it obtained the top-ranked performance among nine systems.
Generating Attractive and Authentic Copywriting from Customer Reviews
NAACL 2024, June 2024
Yu-Xiang Lin, Wei-Yun Ma
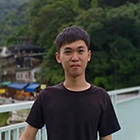
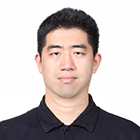
Abstract
The goal of product copywriting is to capture the interest of users and enhance their experience by emphasizing the features of products through text descriptions. As e-commerce platforms offer a wide range of services, it's becoming essential to dynamically adjust the styles of these auto-generated descriptions. Traditional approaches to copywriting generation often rely solely on specified product attributes, which may result in dull and repetitive content. To tackle this issue, we propose to generate copywriting based on customer reviews, as they provide firsthand practical experiences with the product, offering a richer source of information than just product attributes. We have developed a sequence-to-sequence framework, enhanced with reinforcement learning, to produce copywriting that is attractive, authentic, and rich in information. Our framework outperforms all existing baseline and zero-shot large language models, including LLaMA-2-chat-7B and GPT-3.5\footnote{In this work, we use gpt-3.5-turbo-0613. }, in terms of both attractiveness and faithfulness. Furthermore, this work features the use of LLMs for aspect-based summaries collection and argument allure assessment. Experiments demonstrate the effectiveness of using LLMs for marketing domain corpus construction. The dataset will be made available in the future.
Plug-in Language Model: Controlling Text Generation with a Simple Regression Model
NAACL 2024, June 2024
Nai-Chi Yang, Wei-Yun Ma, Pu-Jen Cheng
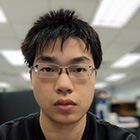
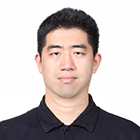
Abstract
Large-scale pre-trained language models have displayed unrivaled capacity in generating text that closely resembles human-written text. Nevertheless, generating texts adhering to specific conditions without fine-tuning or adding new parameters can be challenging.
Contemporary approaches commonly rely on either prompts or auxiliary models to avoid modifying the language models. These auxiliary models are designed to assess whether a generated token contributes to meeting the desired requirements. These approaches adjust the distribution of the next token during the inference phase by leveraging the prediction score of the desired attribute to calculate gradients. However, these auxiliary models typically require the language model's latent states. This prerequisite challenges integrating various existing black box attribute models or tools.
We present the Plug-in Language Model (PiLM) as a solution to address the limitations. PiLM leverages reinforcement learning to utilize black box tools directly, adjusting the latent state to control text generation. However, performing backpropagation during the inference phase is time-consuming for PiLM. By replacing backpropagation with a simple regression model, PiLM can achieve an inference time comparable to that of the original LLM.
Experiment results show that our approaches in this paper outperform existing state-of-the-art methods that rely on gradient-based, weighted decoding, or prompt-based methodologies.
Automatic Construction of a Chinese Review Dataset for Aspect Sentiment Triplet Extraction via Iterative Weak Supervision
LREC-COLING 2024, May 2024
Chia-Wen Lu, Ching-Wen Yang, Wei-Yun Ma
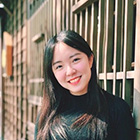
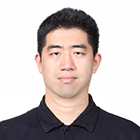
Abstract
Aspect Sentiment Triplet Extraction (ASTE), introduced in 2020, is a task that involves the extraction of three key elements: target aspects, descriptive opinion spans, and their corresponding sentiment polarity. This process, however, faces a significant hurdle, particularly when applied to Chinese languages, due to the lack of sufficient datasets for model training, largely attributable to the arduous manual labeling process. To address this issue, we present an innovative framework that facilitates the automatic construction of ASTE via Iterative Weak Supervision, negating the need for manual labeling, aided by a discriminator to weed out subpar samples. The objective is to successively improve the quality of this raw data and generate supplementary data. The effectiveness of our approach is underscored by our results, which include the creation of a substantial Chinese review dataset. This dataset encompasses over 60,000 Google restaurant reviews in Chinese and features more than 200,000 extracted triplets. Moreover, we have also established a robust baseline model by leveraging a novel method of weak supervision. Both our dataset and model are openly accessible to the public.
DTC: A Drift-Tolerant Coding to Improve the Performance and Energy Efficiency of Multi-Level-Cell Phase-Change Memory
IEEE Transactions on Computer-Aided Design of Integrated Circuits and Systems (TCAD), October 2023
Yi-Shen Chen, Yuan-Hao Chang, and Tei-Wei Kuo
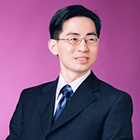
Abstract
Recently, phase-change memory (PCM) has emerged as a promising memory and storage technology. By storing multiple bits in a PCM cell, multi-level-cell (MLC) PCM further reduces the per-bit cost to improve its competitiveness. However, MLC PCM suffers from the high write latency and energy consumption caused by its complex write operations. Different from the existing works that attempt to improve the write latency and energy efficiency of the physical program & verify strategy for MLC PCM, we propose DTC, a drift-tolerant coding scheme, to apply fast write operation on MLC PCM without sacrificing the data accuracy. By exploiting the resistance drift and asymmetric write characteristics of PCM cells, the proposed DTC can significantly reduce the write latency and energy consumption of MLC PCM. Meanwhile, we propose a segmentation strategy to further improve the write performance with our coding scheme and an elimination methodology to avoid issuing unnecessary update operations. A series of analyses and experiments was conducted to evaluate the capability of the proposed scheme. It is encouraging that the proposed scheme can reduce 16.8%–32.1% energy consumption and 20.1%–32.6% write latency under the representative benchmarks, compared with the existing well-known schemes.
TRAIN: A Reinforcement Learning Based Timing-Aware Neural Inference on Intermittent Systems
ACM/IEEE International Conference on Computer-Aided Design (ICCAD), October 2023
Shu-Ting Cheng, Wen Sheng Lim, Chia-Heng Tu and Yuan-Hao Chang
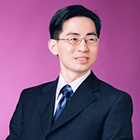
Abstract
Intermittent systems become popular to be considered as the solutions of various application domains, thanks to the maturation of energy harvesting technology. Environmental monitoring is such an example and it is a time-sensitive application domain. In order to report the perceived environmental status in a timely manner, methods have been proposed to consider the freshness of the collected information on such systems with unstable power sources. Nevertheless, these methods cannot be applied to neural network workloads since these methods do not consider the delivered model accuracy. On the other hand, while there have been studies for deploying neural network applications on intermittent systems, they depend on branchy network architectures, each branch representing an energy-accuracy tradeoff, and do not take into account a time constraint, which tends to cause system failures because of the frequent generation of expired data. In this work, the first timing-aware framework TRAIN is proposed to deploy the neural network models on the intermittent systems by considering energy, time constraint, and delivered model accuracy. Compared with the prior studies that depend on branchy network architectures, TRAIN offers a broadened solution space representing various energy/time/accuracy tradeoffs. It is achieved by allowing to choose among different implementations of each model layer during the model inference at runtime, and the smart choices are made by the proposed reinforcement learning algorithm. Our results demonstrate TRAIN outperforms the prior study by 65%, regarding the delivered model accuracy. We believe that TRAIN paves the way for building complex applications on intermittent systems.
Multi-Task Pseudo-Label Learning for Non-Intrusive Speech Quality Assessment Model
IEEE Int. Conf. Acoustics, Speech, and Signal Processing (ICASSP2024), April 2024
Ryandhimas Zezario, Bo-Ren Brian Bai, Chiou-Shann Fuh, Hsin-Min Wang, and Yu Tsao
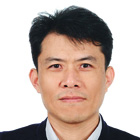
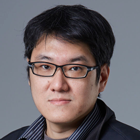
Abstract
This study proposes a multi-task pseudo-label learning (MPL)-based non-intrusive speech quality assessment model called MTQ-Net. MPL consists of two stages: obtaining pseudolabel scores from a pretrained model and performing multitask learning. The 3QUEST metrics, namely Speech-MOS (S-MOS), Noise-MOS (N-MOS), and General-MOS (GMOS), are the assessment targets. The pretrained MOSA-Net model is utilized to estimate three pseudo labels: perceptual evaluation of speech quality (PESQ), short-time objective intelligibility (STOI), and speech distortion index (SDI). Multi-task learning is then employed to train MTQ-Net by combining a supervised loss (derived from the difference between the estimated score and the ground-truth label) and a semi-supervised loss (derived from the difference between the estimated score and the pseudo label), where the Huber loss is employed as the loss function. Experimental results first demonstrate the advantages of MPL compared to training a model from scratch and using a direct knowledge transfer mechanism. Second, the benefit of the Huber loss for improving the predictive ability of MTQ-Net is verified. Finally, the MTQ-Net with the MPL approach exhibits higher overall predictive power compared to other SSL-based speech assessment models.
Is Explanation the Cure? Misinformation Mitigation in the Short-term and Long-term
in Proceedings of The 2023 Conference on Empirical Methods in Natural Language Processing (EMNLP 2023), December 2023
Yi-Li Hsu, Shih-Chieh Dai, Aiping Xiong, Lun-Wei Ku
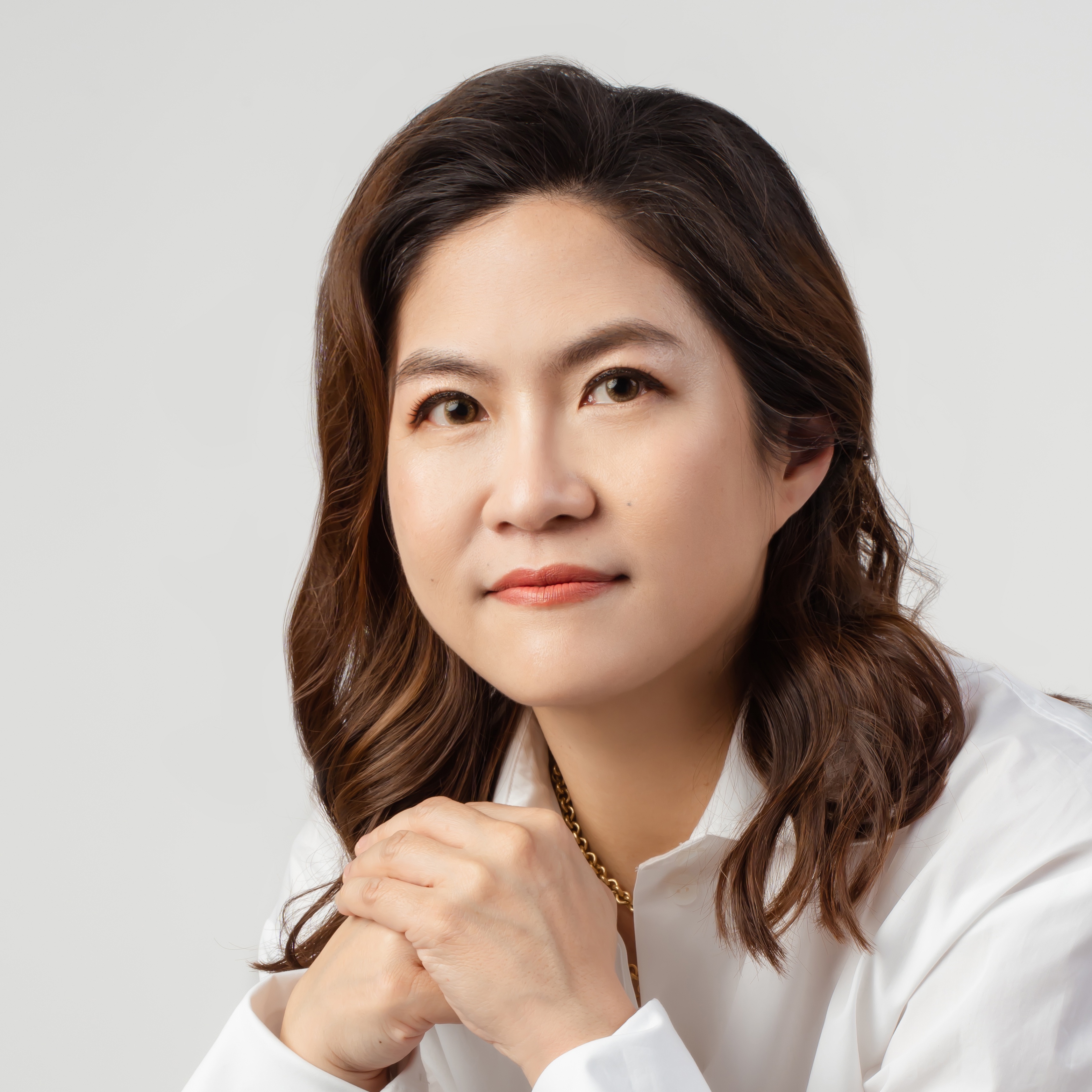
Abstract
With advancements in natural language processing (NLP) models, automatic explanation generation has been proposed to mitigate misinformation on social media platforms in addition to adding warning labels to identified fake news. While many researchers have focused on generating good explanations, how these explanations can really help humans combat fake news is under-explored. In this study, we compare the effectiveness of a warning label and the state-of- the-art counterfactual explanations generated by GPT-4 in debunking misinformation. In a two-wave, online human-subject study, participants (N = 215) were randomly assigned to a control group in which false contents are shown without any intervention, a warning tag group in which the false claims were labeled, or an explanation group in which the false contents were accompanied by GPT-4 generated explanations. Our results show that both interventions significantly decrease participants’ self-reported belief in fake claims in an equivalent manner for the short-term and long-term. We discuss the implications of our findings and directions for future NLP-based misinformation debunking strategies.
LLM-in-the-loop: Leveraging Large Language Model for Thematic Analysis
in Proceedings of The 2023 Conference on Empirical Methods in Natural Language Processing (EMNLP 2023), December 2023
Shih-Chieh Dai, Aiping Xiong, Lun-Wei Ku
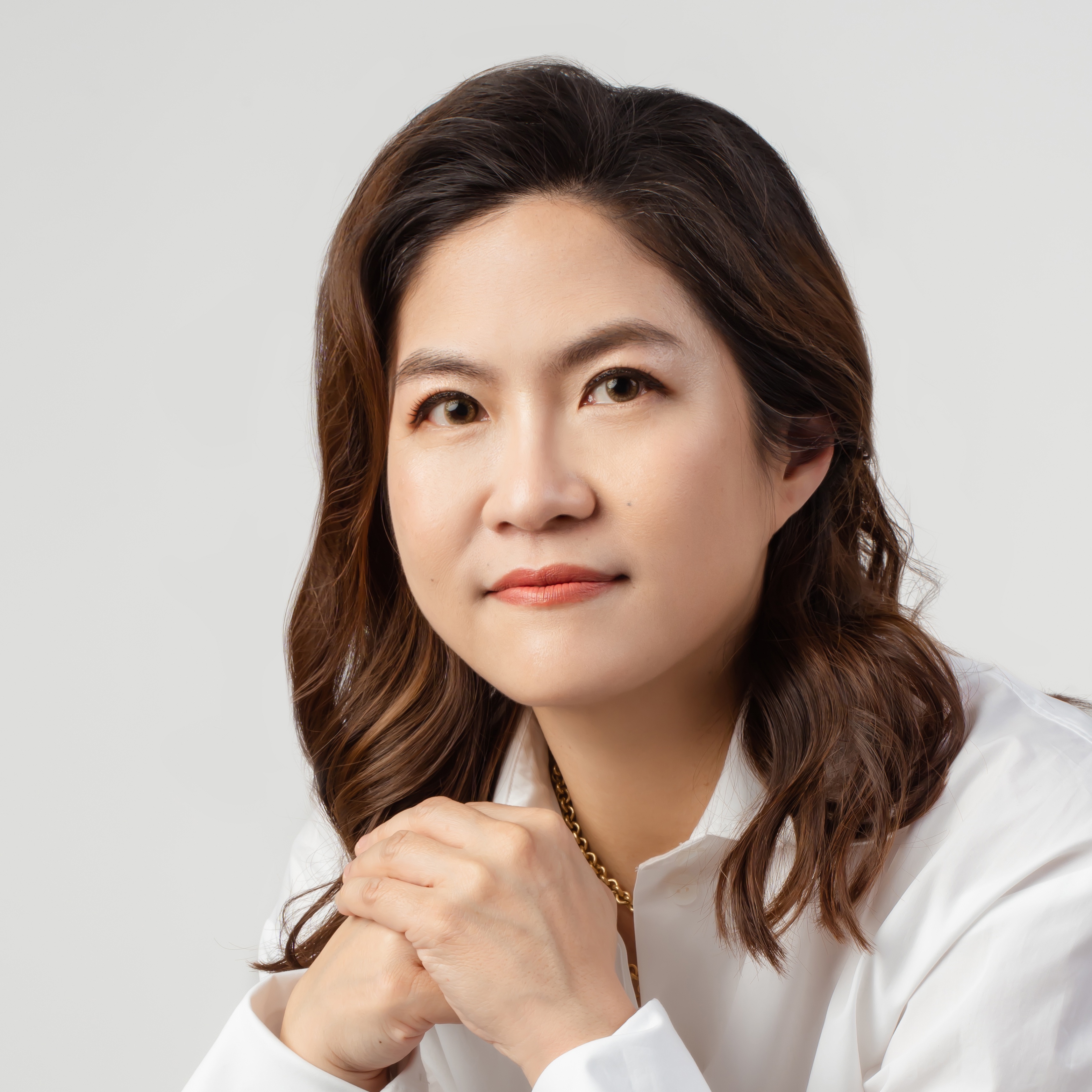
Abstract
Thematic analysis (TA) has been widely used for analyzing qualitative data in many disciplines and fields. To ensure reliable analysis, the same piece of data is typically assigned to at least two human coders. Moreover, to produce meaningful and useful analysis, human coders develop and deepen their data interpretation and coding over multiple iterations, making TA labor-intensive and time-consuming. Recently the emerging field of large language models (LLMs) research has shown that LLMs have the potential replicate human-like behavior in various tasks: in particular, LLMs outperform crowd workers on text-annotation tasks, suggesting an opportunity to leverage LLMs on TA. We propose a human–LLM collaboration framework (i.e., LLM-in-the-loop) to conduct TA with in-context learning (ICL). This framework provides the prompt to frame discussions with a LLM (e.g., GPT-3.5) to generate the final codebook for TA. We demonstrate the utility of this framework using survey datasets on the aspects of the music listening experience and the usage of a password manager. Results of the two case studies show that the proposed framework yields similar coding quality to that of human coders but reduces TA’s labor and time demands.
Location-Aware Visual Question Generation with Lightweight Models
in Proceedings of The 2023 Conference on Empirical Methods in Natural Language Processing (EMNLP 2023), December 2023
Nicholas Collin Suwono, Justin Chen, Tun Min Hung, Ting-Hao Kenneth Huang, I-Bin Liao, Yung-Hui Li, Lun-Wei Ku, Shao-Hua Sun
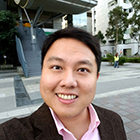
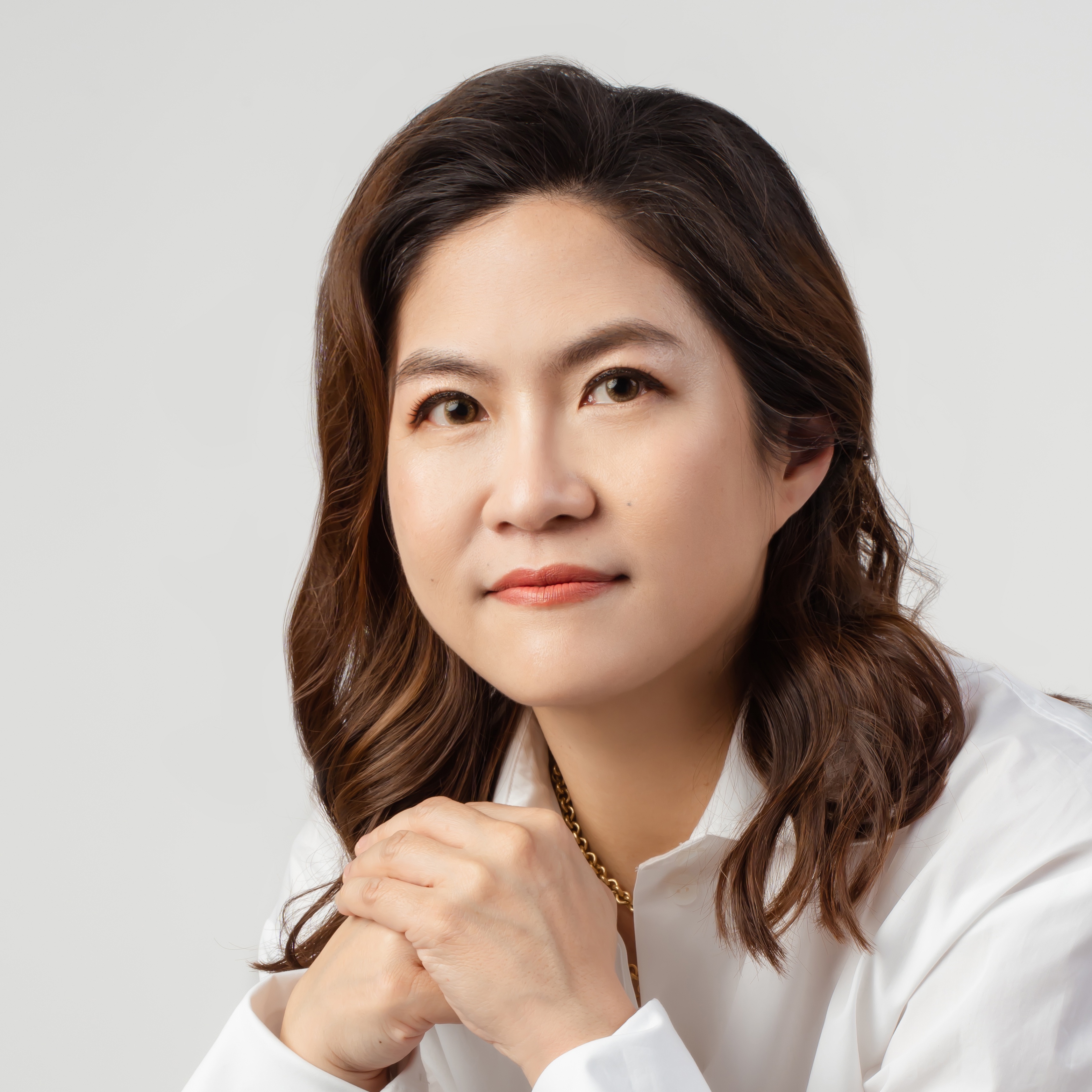
Abstract
This work introduces a novel task, location-aware visual question generation (LocaVQG), which aims to generate engaging questions from data relevant to a particular geographical location. Specifically, we represent such location-aware information with surrounding images and a GPS coordinate. To tackle this task, we present a dataset generation pipeline that leverages GPT-4 to produce diverse and sophisticated questions. Then, we aim to learn a lightweight model that can address the LocaVQG task and fit on an edge device, such as a mobile phone. To this end, we propose a method which can reliably generate engaging questions from location-aware information. Our proposed method outperforms baselines regarding human evaluation (e.g., engagement, grounding, coherence) and automatic evaluation metrics (e.g., BERTScore, ROUGE-2). Moreover, we conduct extensive ablation studies to justify our proposed techniques for both generating the dataset and solving the task.
A formal treatment of bidirectional typing
33rd European Symposium on Programming (ESOP 2024), April 2024
Chen, Liang-Ting and Ko, Hsiang-Shang
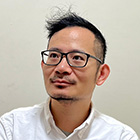
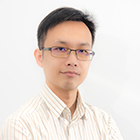
Abstract
There has been much progress in designing bidirectional type systems and associated type synthesis algorithms, but mainly on a case-by-case basis. To remedy the situation, this paper develops a general and formal theory of bidirectional typing, and, as a by-product of our formalism, provides a verified generator of proof-relevant type synthesisers for simply typed languages: for every signature that specifies a mode-correct bidirectionally typed language, there exists a proof-relevant type synthesiser that for an input abstract syntax tree constructs a typing derivation if any, gives its refutation if not, or reports that the input does not have enough type annotations. Soundness, completeness, and mode-correctness are studied universally for all signatures, which are sufficient conditions for deriving a type synthesiser. We propose a preprocessing step called mode decoration, which helps the user to deal with missing type annotations in a given abstract syntax tree. The entire development is formalised in Agda and can be further integrated with other language-formalisation frameworks.
Game Solving with Online Fine-Tuning
The Thirty-seventh Conference on Neural Information Processing Systems (NeurIPS), December 2023
Ti-Rong Wu, Hung Guei, Ting Han Wei, Chung-Chin Shih, Jui-Te Chin, I-Chen Wu

Abstract
Game solving is a similar, yet more difficult task than mastering a game. Solving a game typically means to find the game-theoretic value (outcome given optimal play), and optionally a full strategy to follow in order to achieve that outcome. The AlphaZero algorithm has demonstrated super-human level play, and its powerful policy and value predictions have also served as heuristics in game solving. However, to solve a game and obtain a full strategy, a winning response must be found for all possible moves by the losing player. This includes very poor lines of play from the losing side, for which the AlphaZero self-play process will not encounter. AlphaZero-based heuristics can be highly inaccurate when evaluating these out-of-distribution positions, which occur throughout the entire search. To address this issue, this paper investigates applying online fine-tuning while searching and proposes two methods to learn tailor-designed heuristics for game solving. Our experiments show that using online fine-tuning can solve a series of challenging 7x7 Killall-Go problems, using only 23.54% of computation time compared to the baseline without online fine-tuning. Results suggest that the savings scale with problem size. Our method can further be extended to any tree search algorithm for problem solving. Our code is available at https://rlg.iis.sinica.edu.tw/papers/neurips2023-online-fine-tuning-solver.
Exploiting Fine-Grained Structured Pruning for Efficient Inference on CNN Model
IEEE International Conference on Parallel and Distributed Systems, December 2023
Cheng-Hung Wu, Ding-Yong Hong, Pangfeng Liu and Jan-Jan Wu

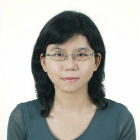
Abstract
Convolutional neural network (CNN) is a deep learning technique that has revolutionized the field of computer vision. In modern CNN models, convolution typically accounts for the majority of the computation time. Model compression is a method used in deep learning to reduce the size of a neural network while preserving its accuracy. Weight pruning removes redundant or unimportant weights from the network. These methods can help reduce the size and computational cost of neural networks while preserving their accuracy. In this work, we propose a dynamic programming algorithm to find a good sparsity ratio for every layer individually under a total time budget based on the execution times and L1 norm of layers. After deciding the sparsity ratio for every layer, we modify TVM to generate code that uses a mask to indicate the data to load for processing. Furthermore, we propose the CHWN layout, where we move the dimension of the batch of data (N) to the innermost dimension to get rid of the varying size in the innermost dimension and make the memory access pattern contiguous. The experiment result shows that our scheme can achieve 0.35% accuracy improvement and a 1.55x speedup on VGG-16 with the ImageNet dataset than the dense model. Convolutional neural network (CNN) is a deep learning technique that has revolutionized the field of computer vision. In modern CNN models, convolution typically accounts for the majority of the computation time. Model compression is a method used in deep learning to reduce the size of a neural network while preserving its accuracy. Weight pruning removes redundant or unimportant weights from the network. These methods can help reduce the size and computational cost of neural networks while preserving their accuracy. In this work, we propose a dynamic programming algorithm to find a good sparsity ratio for every layer individually under a total time budget based on the execution times and L1 norm of layers. After deciding the sparsity ratio for every layer, we modify TVM to generate code that uses a mask to indicate the data to load for processing. Furthermore, we propose the CHWN layout, where we move the dimension of the batch of data (N) to the innermost dimension to get rid of the varying size in the innermost dimension and make the memory access pattern contiguous. The experiment result shows that our scheme can achieve 0.35% accuracy improvement and a 1.55x speedup on VGG-16 with the ImageNet dataset than the dense
LC4SV: A Denoising Framework Learning to Compensate for Unseen Speaker Verification Models
IEEE Workshop on Automatic Speech Recognition and Understanding (ASRU 2023), December 2023
Chi-Chang Lee, Hong-Wei Chen, Chu-Song Chen, Hsin-Min Wang, Tsung-Te Liu, and Yu Tsao
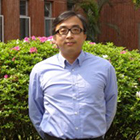
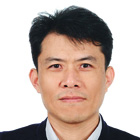
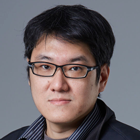
Abstract
The performance of speaker verification (SV) models may drop dramatically in noisy environments. A speech enhancement (SE) module can be used as a front-end strategy. However, existing SE methods may fail to bring performance improvements to downstream SV systems due to artifacts in the predicted signals of SE models. To compensate for artifacts, we propose a generic denoising framework named LC4SV, which can serve as a pre-processor for various unknown downstream SV models. In LC4SV, we employ a learning-based interpolation agent to automatically generate the appropriate coefficients between the enhanced signal and its noisy input to improve SV performance in noisy environments. Our experimental results demonstrate that LC4SV consistently improves the performance of various unseen SV systems. To the best of our knowledge, this work is the first attempt to develop a learning-based interpolation scheme aiming at improving SV performance in noisy environments.
The VoiceMOS Challenge 2023: Zero-shot Subjective Speech Quality Prediction for Multiple Domains
IEEE Workshop on Automatic Speech Recognition and Understanding (ASRU 2023), December 2023
Erica Cooper, Wen-Chin Huang, Yu Tsao, Hsin-Min Wang, Tomoki Toda, and Junichi Yamagishi
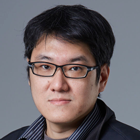
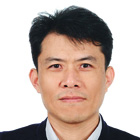
Abstract
We present the second edition of the VoiceMOS Challenge, a scientific event that aims to promote the study of automatic prediction of the mean opinion score (MOS) of synthesized and processed speech. This year, we emphasize real-world and challenging zero-shot out-of-domain MOS prediction with three tracks for three different voice evaluation scenarios. Ten teams from industry and academia in seven different countries participated. Surprisingly, we found that the two sub-tracks of French text-to-speech synthesis had large differences in their predictability, and that singing voice-converted samples were not as difficult to predict as we had expected. Use of diverse datasets and listener information during training appeared to be successful approaches.
Audio-Visual Mandarin Electrolaryngeal Speech Voice Conversion
Interspeech2023, August 2023
Yung-Lun Chien, Hsin-Hao Chen, Ming-Chi Yen, Shu-Wei Tsai, Hsin-Min Wang, Yu Tsao, and Tai-Shih Chi
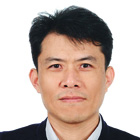
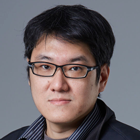
Abstract
Electrolarynx is a commonly used assistive device to help patients with removed vocal cords regain their ability to speak. Although the electrolarynx can generate excitation signals like the vocal cords, the naturalness and intelligibility of electrolaryngeal (EL) speech are very different from those of natural (NL) speech. Many deep-learning-based models have been applied to electrolaryngeal speech voice conversion (ELVC) for converting EL speech to NL speech. In this study, we propose a multimodal voice conversion (VC) model that integrates acoustic and visual information into a unified network. We compared different pre-trained models as visual feature extractors and evaluated the effectiveness of these features in the ELVC task. The experimental results demonstrate that the proposed multimodal VC model outperforms single-modal models in both objective and subjective metrics, suggesting that the integration of visual information can significantly improve the quality of ELVC.
A Training and Inference Strategy Using Noisy and Enhanced Speech as Target for Speech Enhancement without Clean Speech
Interspeech2023, August 2023
Li-Wei Chen, Yao-Fei Cheng, Hung-Shin Lee, Yu Tsao, and Hsin-Min Wang
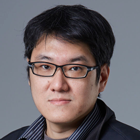
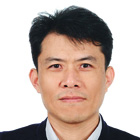
Abstract
The lack of clean speech is a practical challenge to the development of speech enhancement systems, which means that there is an inevitable mismatch between their training criterion and evaluation metric. In response to this unfavorable situation, we propose a training and inference strategy that additionally uses enhanced speech as a target by improving the previously proposed noisy-target training (NyTT). Because homogeneity between in-domain noise and extraneous noise is the key to the effectiveness of NyTT, we train various student models by remixing 1) the teacher model’s estimated speech and noise for enhanced-target training or 2) raw noisy speech and the teacher model’s estimated noise for noisy-target training. Experimental results show that our proposed method outperforms several baselines, especially with the teacher/student inference, where predicted clean speech is derived successively through the teacher and final student models.
Function Clustering to Optimize Resource Utilization on Container Platform
IEEE International Conference on Parallel and Distributed Systems, December 2023
Chao-Yu Lee, Ding-Yong Hong, Pangfeng Liu and Jan-Jan Wu

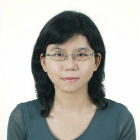
Abstract
In recent years, container technology has gained significant attention in the software industry, with many businesses opting for its elasticity, cost-effectiveness, is not without challenges. The ”cold-start” problem is the most critical issue in deploying containers. The cold-start time is the delay from a container being provisioned on a physical server to getting ready to run the application. The end users need to endure delays when they run the application at the time the container has just started. These delays cause a negative user experience and may deteriorate the business’s profitability. The most common way to ensure a seamless user experience is to keep a substantial number of containers active throughout the day, which causes resource over-provision. Conversely, closing the container right after handling the requests can reduce memory consumption but generate a cold start whenever the request arrives. Cold start occurrence and resource usage is a trade-off and presents a significant challenge on the container platform. To address this challenge, we observe that serving consecutive requests with the same container can notably decrease the number of cold starts. We propose TAC, a Temporal Adjacency Function Clustering algorithm, to meet the challenge. TAC selects the functions with time adjacency requests into a cluster from the historical data. TAC packs functions serving time adjacency requests into a cluster to reduce cold starts and enable efficient resource utilization. The experiment result shows that TAC reduces 8% cold start occurrences and 53% memory usage with the real-world traces compared to the state-of-the-art methods, e.g., Defuse and Hybrid histogram policy.
Enabling Highly-Efficient DNA Sequence Mapping via ReRAM-based TCAM
ACM/IEEE International Symposium on Low Power Electronics and Design (ISLPED), August 2023
Yu-Shao Lai, Shuo-Han Chen, and Yuan-Hao Chang

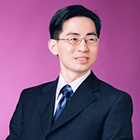
Abstract
In the post-pandemic era, third-generation DNA sequencing (TGS) has received increasing attention from both academics and industries. As TGS technologies have become a requisite for extracting DNA sequences, the DNA sequence mapping, which is the most basic bioinformatics application and the core of polymerase chain reaction (PCR) tests, receives great challenges, due to the large size and noisy nature of TGS technologies. In addition, the ever-increasing data volume of DNA sequences also induces the issue of memory wall while large datasets are moved between the memory and the computing units. However, much less effort has been devoted to DNA sequence mapping acceleration while considering both the memory wall issue and the challenges of TGS technologies. To enable highly-efficient DNA sequence mapping, this study proposes a novel resistive random-access memory (ReRAM)-based ternary content-addressable memory (TCAM) and exploits the intrinsic parallelity of ReRAM crossbar for efficient mapping acceleration. Promising results have been demonstrated through a series of experiments with different scales of datasets.