BIC-Based Speaker Segmentation Using Divide-and-Conquer Strategies with Application to Speaker Diarization
IEEE Transactions on Audio, Speech, and Language Processing 18 (2010): 141-157.
Shih-Sian Cheng, Hsin-Min Wang, and Hsin-Chia Fu
- Institute of Information Science, Academia Sinica
The goal of speaker (audio) segmentation is to detect speaker (acoustic) change boundaries in an audio stream. In the last decade, researchers in the speech processing community have expended a great deal of effort on this problem because of its application to many speech and audio processing tasks, such as audio classification, automatic transcription of audio recordings, speaker tracking, and speaker diarization. In this paper, we propose three divide-and-conquer approaches for BIC-based speaker segmentation, namely, SeqDACDec1, SeqDACDec2, and DACDec3. The approaches detect speaker changes by recursively partitioning a large analysis window into two sub-windows and recursively verifying the merging of two adjacent audio segments using ΔBIC, a widely adopted distance measure of two audio segments. We compare our approaches to three popular distancebased approaches, namely, Chen and Gopalakrishnan’s window-growing-based approach (WinGrow), Siegler et al.’s fixedsize sliding window approach (FixSlid), and Delacourt and Wellekens’s DISTBIC approach (DISTBIC_pR), by performing computational cost analysis and conducting speaker change detection experiments on two broadcast news data sets. The results show that the proposed approaches are more efficient and achieve higher segmentation accuracy than the compared distance-based approaches. In addition, we apply the segmentation approaches discussed in this paper to the speaker diarization task. The experimental results show that a more effective segmentation approach leads to better diarization accuracy.
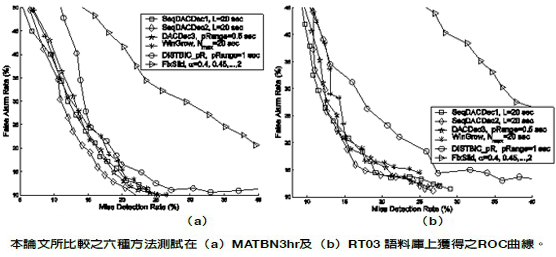
Clustering Complex Data with Group-Dependent Feature Selection
Lecture Notes in Computer Science 6316 (2010): 84-97.
Yen-Yu Lin, Tyng-Luh Liu, Chiou-Shann Fuh
- Institute of Information Science, Academia Sinica
We propose a clustering approach with the emphasis on detecting coherent structures in a complex dataset, and illustrate its effectiveness with computer vision applications. By complex data, we mean the attribute variations among the data are too extensive such that clustering based on a single feature representation/descriptor is insufficient to faithfully divide the data into meaningful groups. Our method thus assumes the data are represented with various feature representations, and aims to uncover the underlying cluster structure. To that end, we associate each cluster with a boosting classifier derived from multiple kernel learning, and apply the cluster-specific classifier to performing feature selection cross various descriptors to best separate data of the cluster from the rest. Specifically, we integrate the multiple, correlative training tasks of the cluster-specific classifiers into the clustering procedure, and cast them as a joint constrained optimization problem. Through the optimization iterations, the cluster structure is gradually revealed by these classifiers, while their discriminant power to capture similar data would be progressively improved owing to better data labeling.
Efficient Simulation of the Spatial Transmission Dynamics of Influenza
PLoS ONE 2010, 5, e13292
Meng-Tsung Tsai, Tsurng-Chen Chern, Jen-Hsiang Chuang, Chih-Wen Hsueh, Hsu-Sung Kuo, Churn-Jung Liau, Steven Riley, Bing-Jie Shen, Chih-Hao Shen, Da-Wei Wang, and *Tsan-Sheng Hsu
- Institute of Information Science, Academia Sinica
As large spatially resolved data sets are constructed to investigate the spreading patterns of pandemic influenza, the need for efficient simulation code becomes clear. Here, we present a significant improvement to the efficiency of an individual based stochastic disease simulation framework commonly used in previous studies. We quantify the performance of the revised algorithm and present an alternative parameterization of the model in terms of the basic reproductive number. We apply the model to the population of Taiwan and demonstrate how the location of the initial seed can influence spatial incidence profiles of the epidemic. The ability to perform efficient simulation allows us to run a batch of simulations and take account of their average in real time. The averaged data are stable and can be used to differentiate spreading patterns that are not readily seen by only conducting a few runs.